AI in Finance: The Rise of Algorithmic Trading and Financial Anal
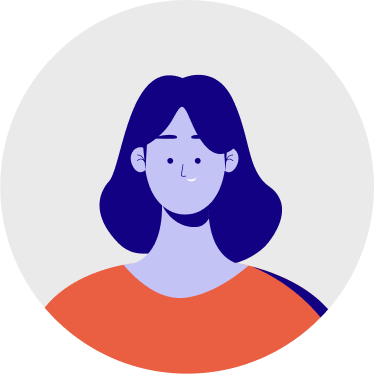
Introduction: The financial industry has always been at the forefront of adopting cutting-edge technologies to gain a competitive edge in the market. In recent years, artificial intelligence (AI) has emerged as a game-changer, revolutionizing various aspects of finance, from investment strategies to risk management. One of the most significant manifestations of AI's impact on finance is the rise of algorithmic trading, where complex algorithms analyze vast amounts of market data to execute trades at high speeds and optimal prices. Additionally, AI-powered financial analysis techniques leverage machine learning and data analytics to uncover insights, predict market trends, and inform investment decisions. In this blog post, we will delve into the evolution of AI in finance, explore the mechanics of algorithmic trading, and examine the role of AI in financial analysis, with real-world examples highlighting its transformative effects on the industry.
- The Evolution of AI in Finance: The journey of AI in finance dates back several decades, marked by significant advancements in technology and computing power. Initially, financial institutions relied on rule-based systems and statistical models to analyze market data and inform investment decisions. However, the limitations of these traditional approaches became increasingly apparent, prompting the adoption of more sophisticated AI techniques.
One pivotal moment in the evolution of AI in finance was the introduction of neural networks, a class of machine learning algorithms inspired by the structure and function of the human brain. Neural networks revolutionized financial analysis by enabling computers to learn from historical data and make predictions based on complex patterns and relationships. This breakthrough paved the way for the development of deep learning techniques, such as convolutional neural networks (CNNs) and recurrent neural networks (RNNs), which excel at processing and analyzing sequential data, such as time series financial data.
Real-world example: Banks like Wells Fargo and JPMorgan Chase utilize neural networks for credit scoring models, assessing borrowers' creditworthiness and making lending decisions based on factors such as credit history, income, and loan repayment behavior.
- Algorithmic Trading: A Game-Changer in Financial Markets: Algorithmic trading, also known as algo trading or automated trading, represents a paradigm shift in financial markets, where trades are executed by computer algorithms based on predefined instructions and parameters. Unlike traditional manual trading methods, which rely on human intuition and emotion, algorithmic trading leverages AI and machine learning to analyze market data, identify trading opportunities, and execute trades with precision and speed.
The benefits of algorithmic trading are manifold. By automating the trading process, algorithmic trading systems can execute trades at millisecond speeds, exploiting fleeting market inefficiencies and arbitrage opportunities that would be impossible for human traders to detect. Moreover, algorithmic trading algorithms can process vast amounts of market data in real-time, making split-second decisions based on predefined trading strategies and risk parameters.
Real-world example: High-frequency trading (HFT) firms like Citadel Securities and Virtu Financial are pioneers in algorithmic trading, executing millions of trades per day across global financial markets. These firms rely on sophisticated AI algorithms to analyze market data, identify trading signals, and execute trades with lightning-fast speed and precision.
- Machine Learning in Financial Analysis: Machine learning, a subset of AI that focuses on building predictive models from data, has emerged as a powerful tool for financial analysis. By leveraging historical market data, machine learning algorithms can identify patterns, trends, and correlations that inform investment decisions and predict future market movements.
One of the key advantages of machine learning in financial analysis is its ability to adapt and learn from new data, continuously refining and improving its predictive capabilities over time. This iterative learning process enables machine learning models to capture complex relationships in financial markets and make accurate predictions with high confidence levels.
Real-world example: Hedge funds like Renaissance Technologies are renowned for their use of machine learning models in financial analysis. Renaissance's flagship fund, the Medallion Fund, employs machine learning algorithms to analyze market data and identify profitable trading opportunities, consistently outperforming traditional investment benchmarks.
- Predictive Analytics for Investment Strategies: Predictive analytics, a branch of AI that focuses on forecasting future outcomes based on historical data and statistical models, plays a crucial role in shaping investment strategies and portfolio management decisions. By analyzing historical market data, economic indicators, and other relevant factors, predictive analytics models can anticipate market trends, identify trading opportunities, and optimize investment allocations.
Real-world example: Quantitative trading firms like Two Sigma Investments utilize predictive analytics models to predict market trends and adjust their trading strategies accordingly. Two Sigma's proprietary predictive analytics platform analyzes vast amounts of market data to identify patterns and correlations, informing its trading decisions and driving superior investment performance.
- Risk Management and Compliance: AI technologies are also transforming risk management and compliance functions within financial institutions, enabling more effective detection and mitigation of financial risks and regulatory compliance requirements. By analyzing large volumes of transactional data, AI-powered risk management systems can identify suspicious activities, detect anomalies, and prevent fraudulent transactions in real-time.
Real-world example: Banks like Goldman Sachs and Bank of America employ AI-driven risk management systems to monitor transactional activities and identify potential risks. These systems utilize advanced machine learning algorithms to analyze transactional data, identify patterns of fraudulent behavior, and flag suspicious transactions for further investigation.
Conclusion: The rise of AI in finance has ushered in a new era of innovation and disruption, transforming the way financial institutions operate, investors make decisions, and markets function. From algorithmic trading systems that execute trades at lightning speed to machine learning models that predict market trends with unprecedented accuracy, AI technologies are reshaping the financial landscape in profound ways. As AI continues to evolve and its applications in finance expand, we can expect to see further innovation and disruption in the industry, with implications for investors, traders, and financial professionals alike. Embracing AI-driven technologies is no longer a choice but a necessity for staying competitive in today's dynamic and rapidly evolving financial markets.